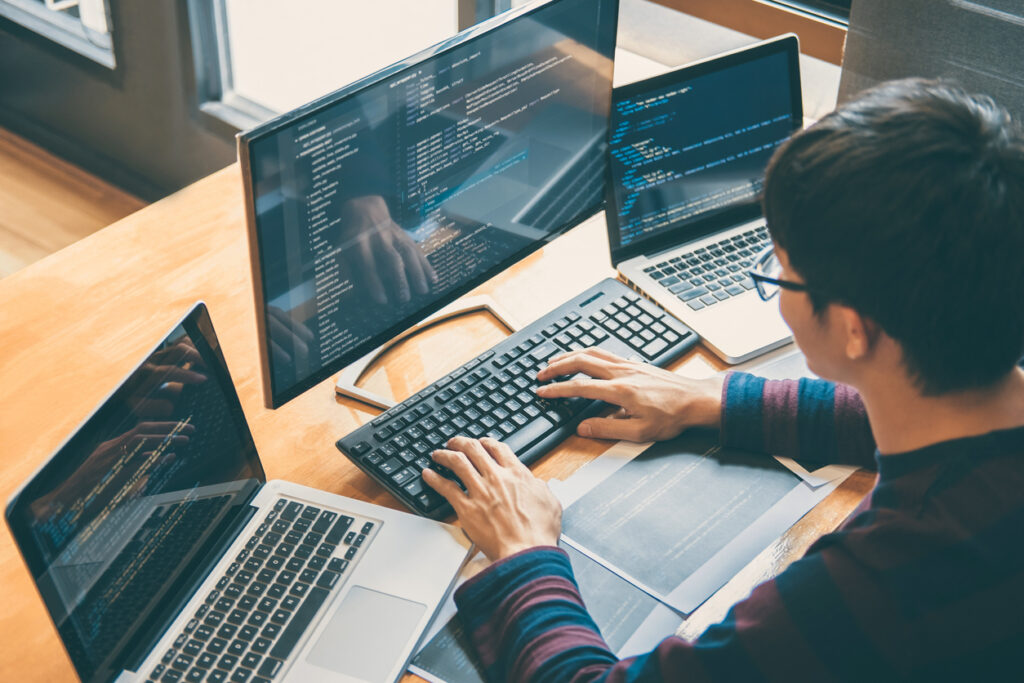
Performance optimization is the process of improving the speed, efficiency, and responsiveness of systems and applications. In today’s competitive IT and business environment, it is essential for meeting user expectations and adapting to evolving demands. However, optimization is not a one-time task. It requires an ongoing commitment to continuous improvement.
Overview
Quality Assurance (QA) teams play a critical role in ensuring system performance by implementing testing strategies that identify issues early in the development process. Through regular evaluations and the use of automated testing tools, QA supports the consistent performance of applications across the software lifecycle. Their input provides developers with actionable insights, encouraging collaboration and enabling iterative improvements. This proactive approach contributes to the long-term efficiency, reliability, and responsiveness of software systems.
This article outlines key strategies for enhancing system performance, focusing on the importance of monitoring performance metrics, optimizing resource utilization, and designing scalable solutions. It highlights the role of automated testing, effective caching, and network optimization, while also underscoring the need for continuous learning and adaptation in response to evolving technologies.
Performance monotoring
Real-time tracking of key metrics
An automated system for monitoring key performance indicators (KPIs) is essential for maintaining visibility into system behavior. Metrics such as system load, response time, throughput, and memory usage provide critical insights. Continuous tracking allows for the timely detection of anomalies, helping to sustain optimal performance and reduce the risk of disruptions.
Defining and using performance benchmarks
Setting well-defined performance benchmarks is important for evaluating system efficiency and identifying areas for improvement. These benchmarks (based on historical trends, industry standards, and organizational goals) establish a baseline for expected performance. Common benchmarks may include response times, processing speeds, or error rates. Comparing real-time performance against these standards supports informed decision-making and ongoing system optimization.
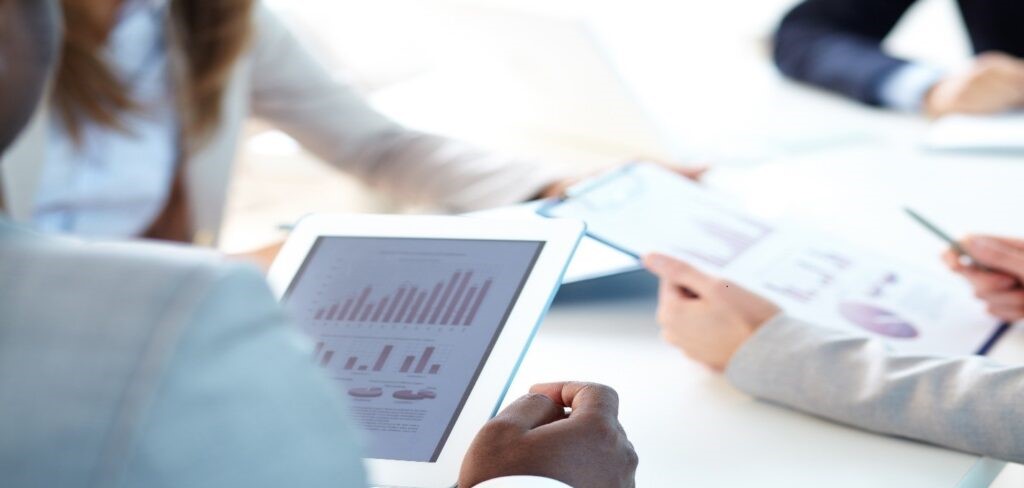
Regular audits and profiling
Conducting performance audits
Routine audits of code, system architecture, and infrastructure are essential for identifying inefficiencies and performance bottlenecks. These audits typically combine manual code reviews by experienced developers with automated analysis using specialized tools.
Manual reviews are effective in detecting logic errors, poor coding practices, and architectural concerns, while automated tools provide detailed diagnostics on performance-related issues. Conducting audits on a regular basis enables proactive maintenance, supporting overall system efficiency, stability, and long-term scalability.
Applying profiling techniques
Profiling tools are instrumental in analyzing application behavior and identifying resource-intensive operations. They help detect performance hotspots, assess memory usage, and guide optimization efforts.
Examples include:
- Chrome DevTools: Built into Google Chrome, this tool assists in debugging front-end code, monitoring network activity, and profiling JavaScript execution to identify rendering delays and performance issues.
- perf (Linux): A low-overhead profiling tool that provides detailed information on CPU cycles, memory usage, and other performance metrics for system-level analysis.
- .NET and Java Profilers: Tools such as Visual Studio Profiler and Java Mission Control deliver deep insights into runtime behavior, helping locate inefficient code paths, memory leaks, and thread contention.
The effective use of these profiling techniques supports the development of high-performance, resource-efficient applications.
Scalability and capacity planning
Scaling strategies
To address system performance and capacity, organizations typically employ horizontal or vertical scaling, depending on application needs and infrastructure design.
- Vertical scaling enhances the resources of existing machines—such as increasing CPU, memory, or storage—making it suitable for workloads requiring more processing power, like large-scale data analysis.
- Horizontal scaling adds additional machines or instances to distribute workloads, improving redundancy and fault tolerance. For example, increasing the number of servers during peak traffic can help maintain stable performance in web applications.
Cloud environments offer greater flexibility in scaling. Services such as AWS Auto Scaling automatically adjust compute resources (e.g., EC2 instances) based on real-time demand, optimizing both performance and cost. Similarly, Kubernetes supports horizontal scaling of containerized applications through dynamic allocation of pods or nodes, using resource-based auto-scaling to maintain service reliability.
Employing the appropriate scaling strategy and tools strengthens system efficiency, resilience, and adaptability under variable workloads.
Effective capacity planning
Planning for future system demands involves analyzing current usage patterns and forecasting resource needs based on historical data. This helps guide infrastructure investments and ensures systems remain responsive as usage scales.
Predictive analysis plays a key role in this process. By applying statistical methods and machine learning to usage data, organizations can forecast trends, anticipate high-demand periods, and identify potential bottlenecks. For instance, recognizing consistent traffic increases during specific periods enables preemptive scaling to avoid performance degradation.
Integrating predictive insights into capacity planning supports the development of scalable, efficient systems that can meet both current and future business requirements.
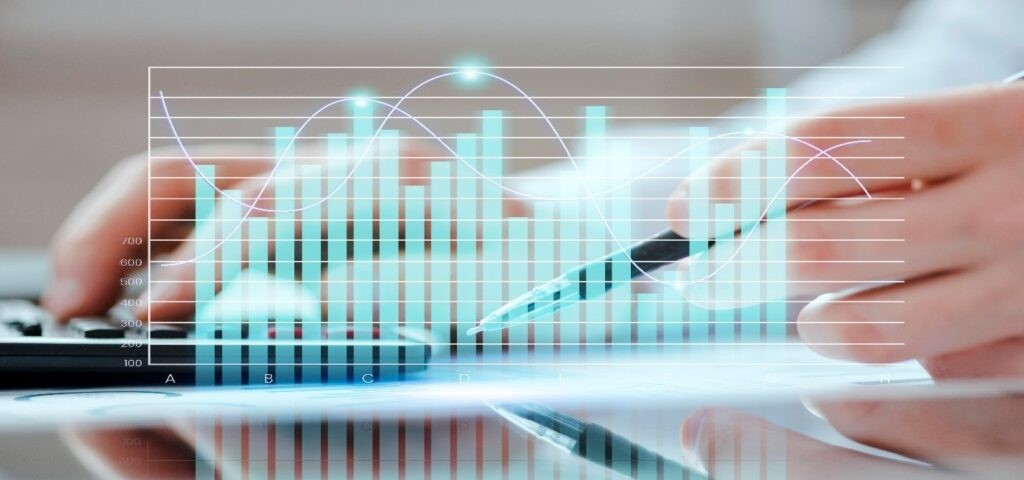
Automating performance testing
As Continuous Integration and Continuous Deployment (CI/CD) practices become standard in modern software development, automated performance testing is increasingly critical for ensuring application quality. Integrating these tests into CI/CD pipelines allows teams to detect performance issues early in the development cycle, minimizing the need for costly remediation later.
Real-time alerting further supports system reliability by notifying teams immediately when performance metrics deviate from expected thresholds. This enables timely intervention and helps prevent minor issues from becoming major disruptions.
Automation also supports workflow efficiency by integrating with project management systems. Performance anomalies can automatically generate tickets, directing issues to the appropriate teams and promoting structured resolution processes. Advanced tools may also provide diagnostic data and remediation suggestions, reducing manual effort and accelerating root cause analysis.
Tools such as Jenkins, GitLab, and Grafana K6 can be incorporated directly into CI/CD pipelines, making performance testing an integral component of the development and deployment lifecycle.
Code and algorithm optimization
The principle of deliberate planning, captured in the phrase “Measure three times, cut once”, is particularly relevant in performance-oriented software development. Thoughtful coding practices and early testing are essential for building scalable systems that can accommodate future growth and evolving requirements.
Code optimization extends beyond writing efficient syntax. It involves aligning application logic and architectural design with long-term performance and scalability objectives. Key practices include removing redundant operations, reducing unnecessary data handling, and minimizing memory usage. Performance-critical tasks such as logging, network communication, and memory allocation should be carefully tuned to avoid introducing bottlenecks.
Routine code refactoring (reorganizing code without altering its external behavior) should be performed incrementally. This approach supports ongoing improvement while reducing the risk of disruption. As systems evolve and new features are introduced, regular refactoring helps maintain code quality and ensures continued alignment with performance goals.
Optimized code contributes significantly to application scalability, reduces technical debt, and enhances system stability. By proactively managing code quality, development teams can reduce the likelihood of complex production issues and deliver a consistent user experience over time.
Database optimization
“Where there is data smoke, there is business fire.”
Thomas Redman
In modern software systems, data plays a central role across all functions, from development and testing to user interaction and support services. Maintaining a high-performing database requires thoughtful design, ongoing optimization, and consistent maintenance. Without these efforts, performance issues can quickly escalate and impact broader business operations.
Before implementing optimization measures, it is essential to understand the nature of the data. Data profiling (analyzing structure, quality, and usage patterns) helps identify inefficiencies and informs a targeted optimization strategy.
Key practices for database performance include:
- Database Scaling: For systems with large volumes of data, horizontal partitioning (sharding) distributes data across multiple servers, while vertical partitioning separates data within a single server. Both approaches reduce the load on individual databases and improve overall responsiveness.
- Query Optimization: Complex or inefficient queries can significantly impact performance, particularly under heavy load. Optimizing queries involves indexing, caching frequently accessed data, and minimizing the retrieval of unnecessary fields to reduce processing overhead.
- Data Archiving: As data accumulates over time, archiving or purging outdated records helps maintain system performance while preserving essential historical information.
- Routine Maintenance: Ongoing tasks such as index defragmentation and configuration tuning are necessary to prevent performance degradation and ensure stable operation.
A well-optimized database is critical to application performance and reliability. By focusing on scalability, query efficiency, and proactive maintenance, organizations can ensure that data remains accessible, timely, and aligned with the needs of the system as it grows.

Caching for improved application performance
Caching is a highly effective, though often underutilized, technique for enhancing application performance. By storing frequently accessed data closer to the point of use, caching significantly reduces data retrieval times, resulting in faster load times and more responsive user interactions.
Common caching strategies include:
- Server-Side Caching: Technologies such as Redis and Memcached allow frequently requested data – session details, product information, or rendered pages – to be stored in memory. This reduces the frequency of database queries, alleviates backend load, and improves system responsiveness.
- Browser Caching: Configuring browsers to locally store static assets such as images, stylesheets, and JavaScript files reduces the need for repeated downloads. This improves performance for returning users and decreases server load.
- Application-Level Caching: Mobile and desktop applications can cache frequently accessed data locally to improve responsiveness, especially in scenarios with limited or unreliable connectivity. For example, retrieving user data from local storage instead of a remote server can significantly enhance performance.
Despite its benefits, caching introduces complexities, particularly around cache invalidation. If not managed correctly, stale data can be served to users, resulting in inconsistencies. To address this, caching strategies should incorporate policies such as time-to-live (TTL), versioning, or manual invalidation mechanisms to ensure data accuracy.
When implemented thoughtfully, caching is a critical component of a broader performance optimization framework, supporting both scalability and a high-quality user experience.
Leveraging cloud and edge computing
Cloud and edge computing have significantly reshaped strategies for application performance, offering scalable and location-aware infrastructure to meet evolving technical demands.
- Cloud Services: Cloud platforms offer flexible, scalable environments that can be tailored to application requirements. Key features such as load balancing distribute incoming traffic across multiple servers, helping to prevent resource saturation and maintain service availability. Autoscaling mechanisms dynamically adjust compute resources based on traffic patterns, ensuring consistent performance while optimizing operational costs.
- Edge Computing: For applications requiring low-latency processing (such as video streaming, IoT systems, or real-time user interfaces) edge computing offers a solution by handling data closer to the user. By processing information at the network’s periphery (e.g., on local edge servers or devices), latency is minimized and response times are improved, particularly for users in remote or distributed regions.
Together, cloud and edge computing enable continuous performance optimization by balancing centralized scalability with localized speed. These approaches allow organizations to deliver responsive, reliable services across diverse user bases and dynamic operating conditions.
Optimizing network performance for efficient data flow
While performance optimization often focuses on code and infrastructure, network efficiency plays a critical role in application responsiveness and reliability. Ensuring optimal data flow requires minimizing latency, improving data transfer speeds, and streamlining routing paths.
- Reducing Latency: Latency (the delay between a user’s action and the system’s response) is especially important in real-time or interactive applications. Techniques such as Anycast routing can improve performance by directing user requests to the nearest available data center, reducing transmission time and improving user experience.
- Content Delivery Networks (CDNs): CDNs, including providers like Cloudflare, Akamai, and Fastly, maintain geographically distributed servers to cache static content closer to end users. By shortening the physical distance between users and content sources, CDNs significantly reduce load times, offload server resources, and improve both performance and scalability.
- Protocol Optimization: Adopting modern network protocols such as HTTP/2 and QUIC can lead to more efficient data transfer. These protocols offer benefits such as multiplexing, reduced round-trip times, and enhanced encryption, contributing to faster and more secure communication.
To maintain optimal performance, it is important to regularly monitor network traffic, identify inefficiencies, and address emerging bottlenecks. A well-optimized network infrastructure supports consistent application performance and contributes to a seamless user experience.
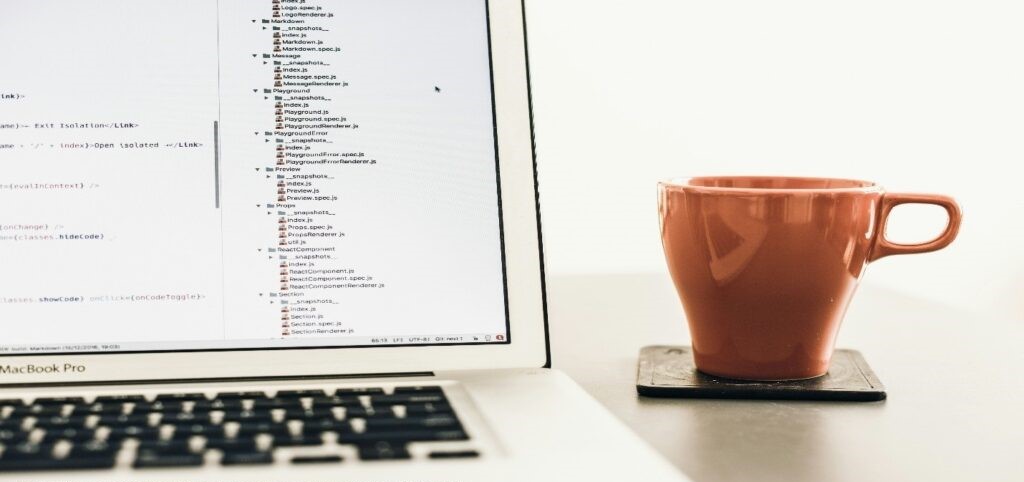
Continuous learning and feedback for sustainable performance
While performance optimization often focuses on code and infrastructure, network efficiency plays a critical role iIn a constantly evolving technological environment, performance optimization must be approached as an ongoing process rather than a one-time initiative. Establishing a culture of continuous learning and feedback is essential to ensure systems remain efficient and responsive over time.
- Staying Informed: As new tools, technologies, and methodologies emerge, it is critical for engineering and IT teams to remain current. This includes engaging with professional communities, attending industry conferences, reviewing technical publications, and participating in knowledge-sharing platforms such as GitHub, Stack Overflow, and specialized forums.
- Establishing Feedback Loops: Effective performance management depends on timely and actionable feedback. Insights from end users, system monitoring tools, and automated performance tests provide early indicators of potential issues. User feedback, in particular, can highlight performance concerns that are not always evident through internal testing.
- Embracing Iterative Improvement: Optimization is a continuous process. Regular audits, incremental updates, and structured evaluations support steady enhancements. Even minor adjustments, whether in application logic, infrastructure, or configurations, can yield significant long-term performance benefits.
By promoting a mindset of continuous improvement, organizations can ensure their systems remain adaptable, efficient, and aligned with evolving user expectations. Integrating learning and feedback into daily workflows reinforces performance optimization as a core operational discipline rather than a reactive measure.
Key takeaways
Continuous performance optimization is essential for organizations seeking to remain competitive in an evolving technological landscape. As user expectations grow and system demands become more complex, sustained performance depends on the consistent application of core practices—such as comprehensive monitoring, automated testing, resource scalability, and efficient data management.
Looking forward, fostering a culture of continuous improvement, informed by real-time feedback and cross-functional collaboration, will be key to maintaining system resilience and responsiveness.
Ultimately, performance optimization should be viewed as an ongoing process. By integrating iterative enhancements into development and operational workflows, organizations can better adapt to future challenges while delivering reliable, high-quality services.
Looking for reliable software development services?
See how we can help.
- Anneke van der Putten
- Call: +31 (0)30 782 0111
- anneke@netrom.nl
- Ronald Bouwmeister
- Call: +31 (0)30 782 0117
- ronald@netrom.nl